

Notiomastodon platensis and Cuvieronius hyodon.
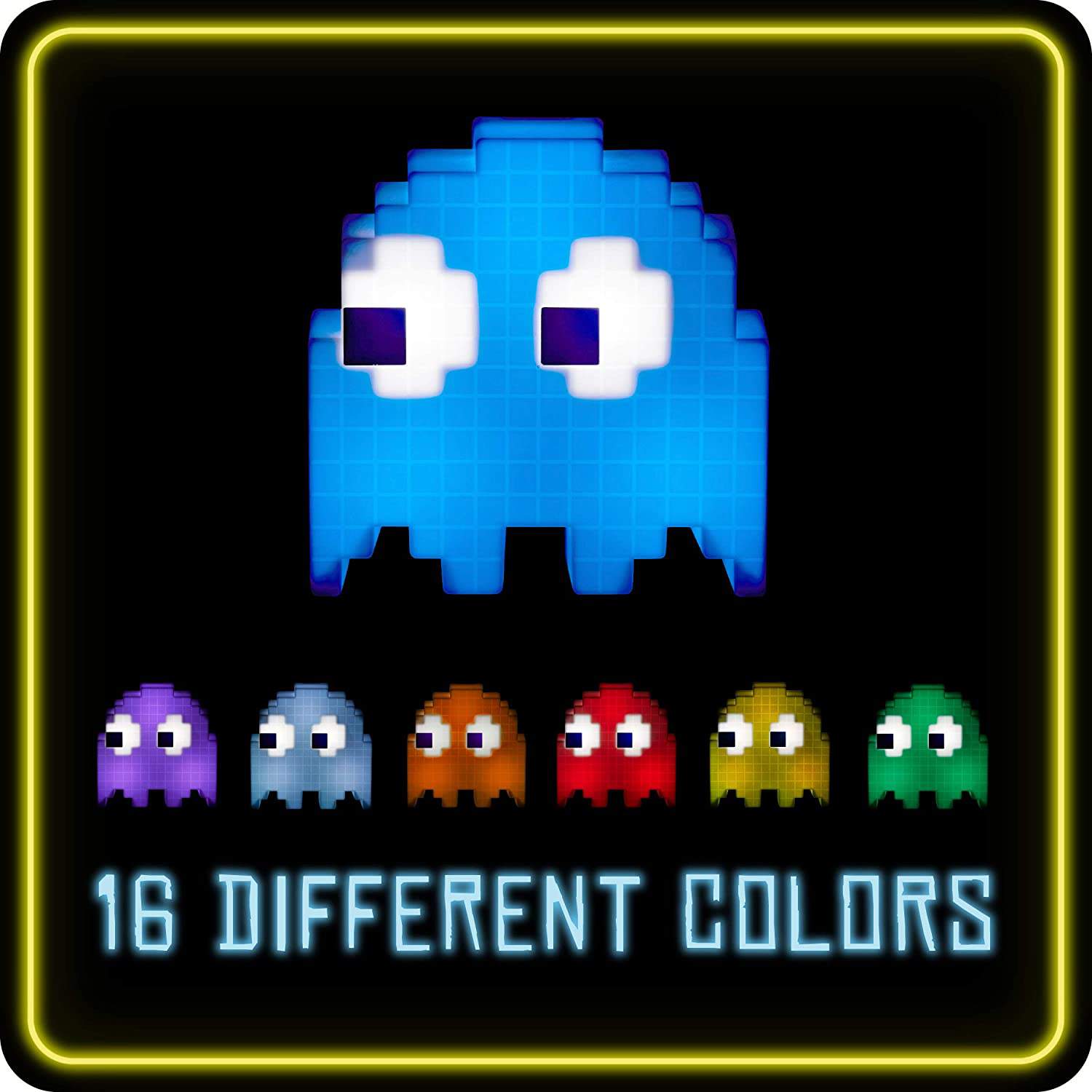
The taxonomy of South American proboscideans is now stable and two species are recognized, Regarding proboscideans in South America subsequent to the publication of Simpson and Paula Couto Studies on South American Gomphotheriidae started around 210 years ago and, 150 years later, theĬlassic study “The mastodonts of Brazil” by Simpson and Paula Couto (1957) attempted to clarify theĬomplex issues related to our understanding of these proboscideans. A clear behavior in this case cannot be concluded, other than the fact that the statistical distribution that fitness variables follow is affected by selection in different directions, that parameters vary in a single generation across them, and that, in general, this kind of behavior will have to be taken into account to adequately address uncertainty in fitness in evolutionary algorithms. Contrary to the usual assumption in this kind of problems, we prove that, in general, the values of two features imply that fitness values do not follow a normal distribution they do present a certain common behavior that changes as evolution proceeds, getting in some cases closer to the standard distribution and in others drifting apart from it. In order to find possible patterns in the statistical behavior of the variables, we track the main features of its distributions, skewness and kurtosis. This work analyzes four different problems related to computational intelligence in videogames, where Evolutionary Computation methods have been applied, and the evaluation of each individual is performed by playing the game, and compare them to other problem, neural network optimization, where performance is also a statistical variable. Thus, it is important to examine the statistical behavior of repeated measurements of performance and, more specifically, the statistical distribution that better fits them. This happens quite often in the context of computational intelligence in games, when either bots behave stochastically, or the target game possesses intrinsic random elements, but it shows up also in other problems as long as there is some random component. In many optimization processes, the fitness or the considered measure of goodness for the candidate solutions presents uncertainty, that is, it yields different values when repeatedly measured, due to the nature of the evaluation process or the solution itself. Results show that (1) the amount of human intervention decreases rapidly, (2) the case base needed to achieve reasonable imitation is considerable smaller than that used in a non-interactive approach (3) the resulting agent outperforms other agents using non-interactive CBR. In this work we describe an interactive and online case-based reasoning system in which the bot gives control to the human player when it reaches game states that are not well represented by cases in its case base, and regains control when the game states are known again. No regret algorithms in online learning settings seem to outperform previous approaches. Although this challenge can be addressed using different Imitation Learning techniques, classic supervised learning approaches do not usually work well due to the violation of the independent and identically distributed assumption for random variables. The goal of these virtual players is to deceive real players and be perceived just as another human player. The imitation of human playing style has been gaining relevance in both the Artificial Intelligence for Games research community and the Digital Game industry over the last decade, achieving a special importance in recent years. Compared with other influence maps, this model could improve the performance of the game AI with time complexity being unchanged. Experiments show this model avoids the weakness of dynamic influence map with location prediction. This method can encode dynamic information into the influence map easily. This model produces different influence values in different direction by adjusting the “distance” between two locations. This paper proposed a dynamic influence map model based on distance adjustment, DADIM. Therefore, the influence produce by the object to a location depends on the relation between the location and the object’s moving direction. When an object moves, it would produce large influence in it’s moving direction than other directions. Some improved IM models can describe dynamic information, but not accurately enough. However, the traditional influence map does not describe dynamic information. Influence map (IM) is often used as a decision supporting technology in game artificial intelligence (AI).
